In contrast to conventional medical procedures and analyses that seek to be general or cover a given demographic, precision medicine focuses on tailored diagnosis, prevention and treatment for individuals. To make this possible, precision medicine techniques make use of an individual’s unique set of traits. These traits may include genomic, lifestyle, physiological and environmental data, all of which combine to create a patient’s “phenotype”.
Why go to the lengths of collecting and organizing all this individual-level data? Many areas of medicine currently rely on coarser or “one size fits all” approaches to diagnosis, treatment and prevention. However, some types of diseases or conditions may be difficult to identify or have complex prognoses that can only be unraveled given more information on a patient’s specific circumstances. One notable example can be found in oncology, where many developments have focused on using phenotype data (primarily genomic markers and indicators) for both diagnosis and treatment (Fig 1).
Though arguably the most well-known application, precision medicine research and applications go beyond bioinformatics and cancer care. Our research focuses on applying machine learning techniques to enhance Intensive care unit (ICU) care. Unlike oncology, where the duration of care may range from days to years, interventions in the ICU must be timely (often in the order of minutes or seconds) to ensure the best patient outcome. As such, our approaches rely on higher resolution and real-time data from vital sign monitors and electronic health records (EHRs).
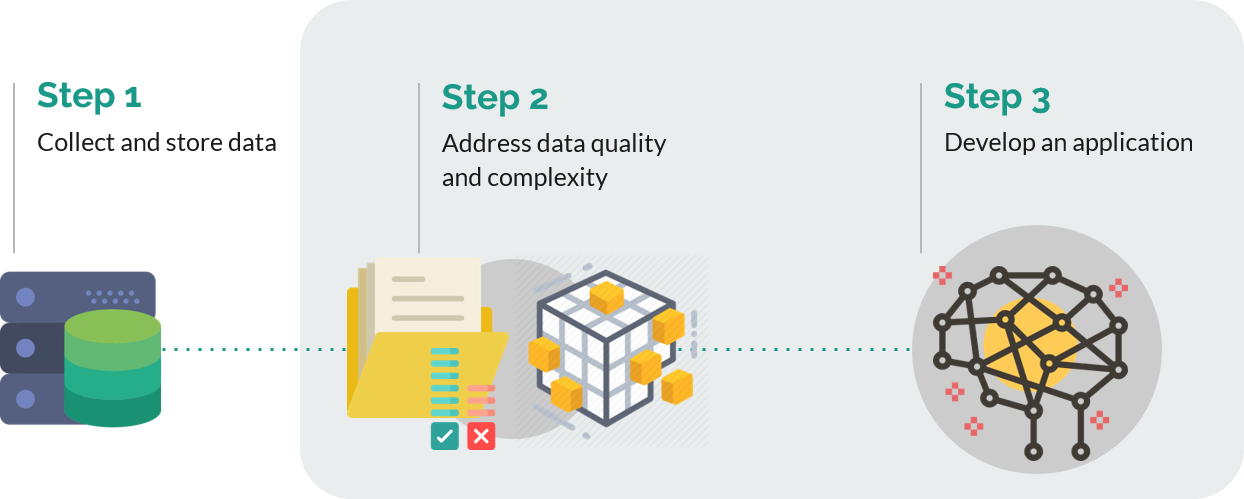
Fig 2. The general process for precision medicine studies. Collecting the data, processing the data to address issues such as quality or the complexity of data available, and then developing a clinically useful application
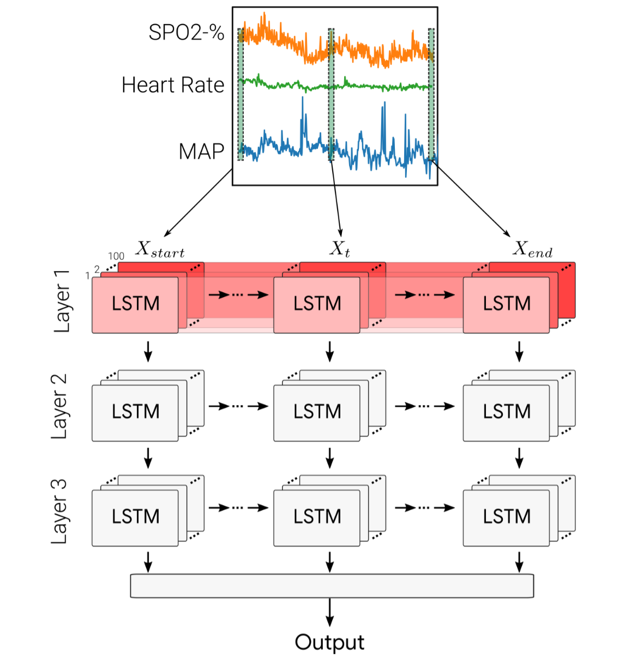
Fig 3. Deep recurrent neural network used to predict acute hypotensive episodes using patient vital signs data.
Our most recent research focused on creating deep learning-based models that can forecast sudden, sustained drops in blood pressure (acute hypotensive episodes/AHEs) using patient vital signs data. Using recurrent neural networks (RNNs) (Fig 3), we were able to model temporal trends in retrospective data in order to predict if a patient will experience an AHE at least 10 minutes in advance. In the ICU, this advance warning would allow providers to proactively intervene for a patient instead of carrying out necessarily riskier and more urgent interventions once an AHE has occurred. We were also able to demonstrate that our deep learning models generalize from institution-specific data to the publicly available MIMIC-III clinical database.
Sample Publications
2020
Chan, Brandon; Chen, Brian; Sedghi, Alireza; Laird, Philip; Maslove, David; Mousavi, Parvin
Generalizable deep temporal models for predicting episodes of sudden hypotension in critically ill patients: a personalized approach Journal Article
In: Scientific Reports, vol. 10, no. 1, pp. 1–10, 2020.
@article{chan2020generalizable,
title = {Generalizable deep temporal models for predicting episodes of sudden hypotension in critically ill patients: a personalized approach},
author = {Brandon Chan and Brian Chen and Alireza Sedghi and Philip Laird and David Maslove and Parvin Mousavi},
year = {2020},
date = {2020-07-10},
journal = {Scientific Reports},
volume = {10},
number = {1},
pages = {1--10},
publisher = {Nature Publishing Group},
keywords = {},
pubstate = {published},
tppubtype = {article}
}
2019
Chan, B; Sedghi, A; Laird, P; Maslove, D; Mousavi, P
Prediction of Patient-specific Acute Hypotensive Episodes in ICU Using Deep Models Proceedings
2019.
@proceedings{552,
title = {Prediction of Patient-specific Acute Hypotensive Episodes in ICU Using Deep Models},
author = {B Chan and A Sedghi and P Laird and D Maslove and P Mousavi},
year = {2019},
date = {2019-01-01},
journal = {The IEEE International Engineering in Medicine and Biology Conference 2019},
keywords = {},
pubstate = {published},
tppubtype = {proceedings}
}
Chan, B; Sedghi, A; Laird, P; Maslove, D; Mousavi, P
Predictive Modeling using Intensive Care Unit Data: Considerations for Data Pre-processing and Analysis Proceedings
2019.
@proceedings{553,
title = {Predictive Modeling using Intensive Care Unit Data: Considerations for Data Pre-processing and Analysis},
author = {B Chan and A Sedghi and P Laird and D Maslove and P Mousavi},
year = {2019},
date = {2019-01-01},
journal = {The IEEE International Engineering in Medicine and Biology Conference},
keywords = {},
pubstate = {published},
tppubtype = {proceedings}
}