Recent advancements in medical imaging technology has provided vast amount of imaging data which can be further used for improving the detection/diagnosis of disease. Our group has been working on various applications of medical image analysis using Machine Learning for the past decade including image classification, predictive modelling, image segmentation, image registration.

Fig 1. Medical imaging provides vast amounts of data that can be analyzed. Image source: https://www.meconferences.com/blog/deep-learning-medical-imaging/
Temporal Enhanced Ultrasound
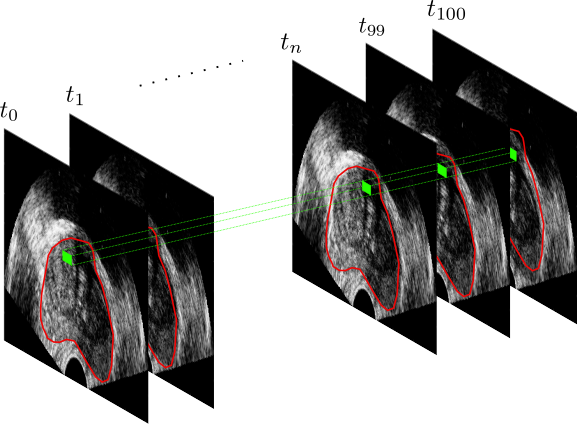
Fig 2. Temporal Enhanced Ultrasound is a set of ultrasound frames captured over a short period of time.
Our group has investigated Temporal Enhanced Ultrasound (TeUS) as a promising imaging modalities for detection of Prostate Cancer (PCa). TeUS is comprised of a set of ultrasound frames captured over a short period of time from a stationary tissue location without mechanical excitation (Fig 2). Our in-vivo and ex-vivo studies have shown tissue-characterizing information of TeUS.
Biopsy is the gold standard for diagnosis of PCa and is often performed under Ultrasound guidance. One of the many challenges with PCa detection is heterogeneity of tissue in each biopsy core which makes the labels for cores extremely noisy. To address this issue in our most recent work, we proposed an unsupervised learning method to learn the similarity of tissue specific TeUS signals with respect to each other. As a result, we generated probability maps of cancer likelihood of each TeUS with respect to each tissue category (Fig 3).

Fig 3. Examples of cancer likelihood maps generated with an unsupervised learning algorithm for cancerous and benign tissues.
Computer Aided Diagnosis
Prostate multi-parametric MRI image interpretation tends to be challenging and the demand for experienced radiologists are increasing every day. Thus, a Computer Assisted Diagnosis (CADx) system can play an important role in assisting radiologists as a second opinion. We investigated 2D and 3D convolutional neural networks for the task of cancer prediction with different combinations of image modalities (Fig 4). We were able to rank 6th among 72 participants in international ProstateX challenge hosted by SPIE, AAPM, NIH, TCIA.
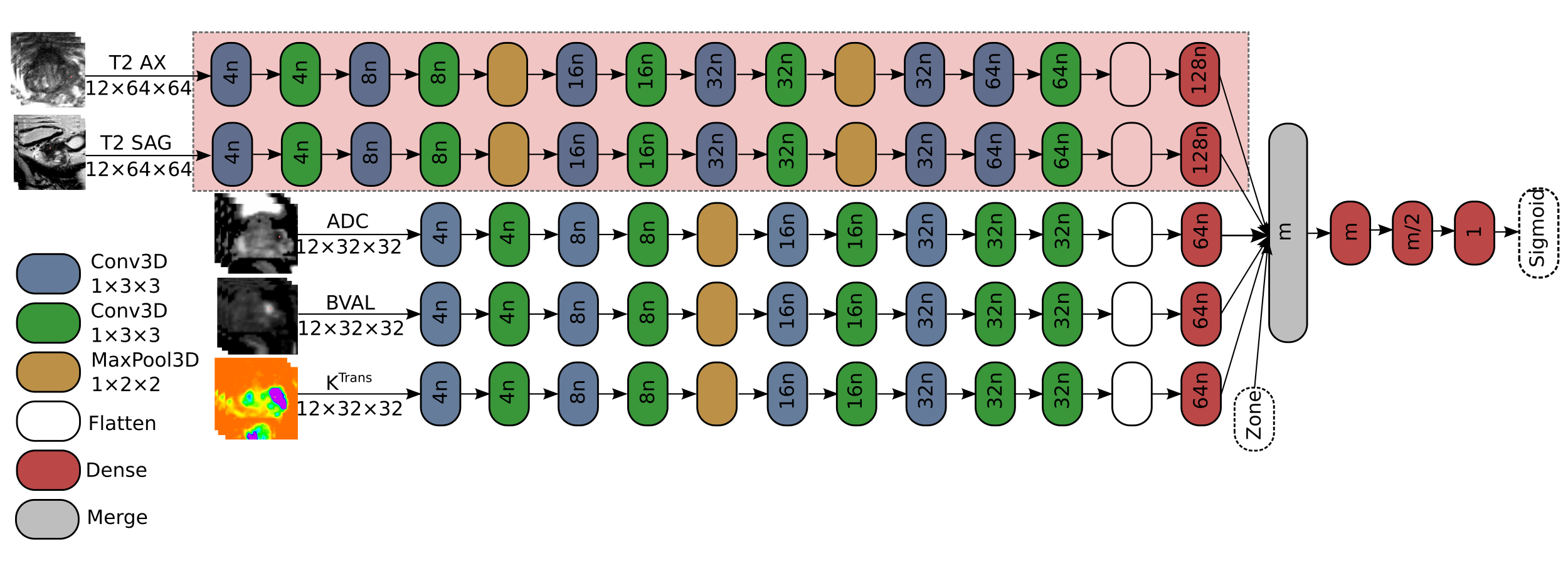
Fig 4. The convolutional neural network designed for the ProstateX challenge.
Image Registration

Fig 5. An example of image registration on a brain CT scan.
Image registration is a fundamental task in every Image-guided Intervention and therapy procedures. Our group has been developing novel probabilistic image registration techniques based on deep classifiers. In a recent study, we trained a multi-class classifiers on discrete displacements of images and utilized it for fast, and accurate registration. In addition, our approach is able to provide uncertainty estimation of the registration results.
Software Development
Artificial Intelligence (AI) is increasingly becoming a tool to enhance various medical image analysis tasks with accuracies comparable to expert clinicians. Integration of AI into the clinical workflow has been slow due to requirements for libraries that are specific to each model, and also environments that are specific to clinical centres. These challenges demonstrate the need for an AI-based solution that can be integrated into any environment with minimum hardware and software overhead. We recently developed a web-based open-source platform, Tesseract-MedicalImaging (Tesseract-MI), which enables deployment of AI models while simultaneously providing standard image viewing and reporting schemes (Fig 6).